How to Simplify Complex Data for Academic Papers
- Lily Taylor
- Feb 7
- 5 min read
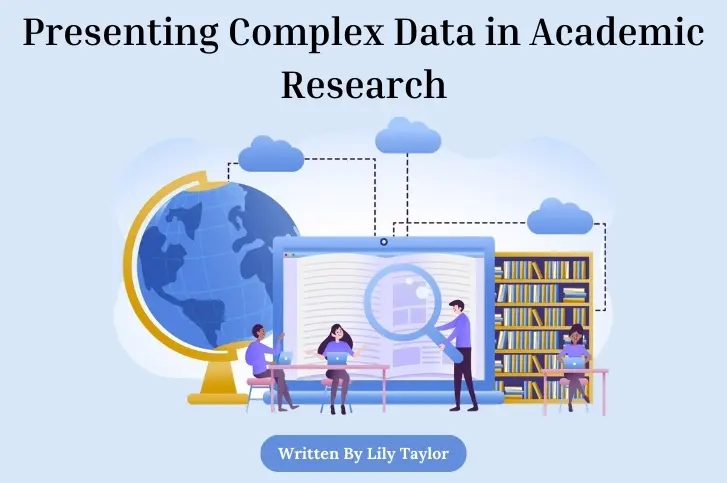
Data serves as the cornerstone of intellectuals in the ever-expanding landscape of academic research. The complex datasets are the ones that are laden with intricate statistics, convoluted variables, and dense numerical frameworks. The consequence of these complex data is that they can bewilder readers. Complex data even can addle readers who are well-versed in scholarly discourse. It is an essential skill for researchers, scholars, students, etc. to simplify complex data without compromising its analytical depth and integrity.
This article is all about the strategic methodology for distilling intricate data into digestible, comprehensible, and impactful insights. The importance of these approaches is that they function to maintain the clarity, coherence, and intellectual sophistication of your academic paper, no matter if you are working with statistical models, qualitative findings, or theoretical approaches.
8 Ways to Simplify Complex Data for Academic Papers
Define the core Message Clearly
First of all, you need to identify the primary message that the data conveys; it should be done before embarking on the journey of simplifying data. For this, you can do the following things:
The data addresses what central question?
Identify the key patterns, trends, or anomalies that emerge from the dataset.
In what ways does the data substantiate or confute the hypotheses?
You can easily eliminate superfluous details and keep your focus on the insights that are the most relevant just by pinpointing the essence of your findings. If you concisely articulate the significance of your data, it would be easy for your readers to grasp the implications of that too without through unnecessary complexity.
Leverage Visual Aids for Clarity
To distil the data into an intuitive and accessible format, visual representations are robust tools, and these are charts, graphs, infographics, and tables. If you transform your raw numbers into meaningful patterns, they can enhance comprehension, engagement, and retention.
How you can choose the right visualisation according to your data presentation demand
Bar graphs are ideal for comparing categories or discrete variables.
Line charts are useful if you want to illustrate trends over time.
Pie charts are used if you want to represent proportions and percentages.
To depict the correlation between variables, you can make use of scatter plots.
In large datasets, the main concern is showcasing density or intensity variations; heat maps are the best.
Strategies that you should bring into practice for the visualisation of data:
To eliminate ambiguity, you should use clear labels, legends, and axes.
The colour scheme that you use should enhance the readability rather than distracting it.
If you want to visualise your data in a way that looks comprehensible, then you should keep it simple and avoid cluttered visuals.
To mention data that are externally obtained, you should always cite the sources.
To simplify the interpretation, you should deploy data visualisations strategically, and it will also reinforce key arguments with compelling visual evidence.
Employ Analogies and Metaphors
Abstract concepts, intricate methodologies, or statistical intricacies are involved in complex data. This may challenge reader comprehension. For unfamiliar data structures to link with relatable real-world phenomena, cognitive bridges are used, which are analogies and metaphors.
Example:
Algorithms trading activities rise 10% and interconnect market validity’s 5% rise.
Statement:
A 10% rise in algorithmic trading activities is correlated with a 5% increase in market volatility.
Simplified with an analogy:
Just as an approaching storm prompts more people to buy umbrellas, rising market volatility triggers increased algorithmic trading.
If you contextualise abstract concepts with familiar frameworks, the analogies transform historic data into intuitive insights.
Break Down Data into digestible Sections
Statistical jargon, percentages, and technical terms are piled on lengthy paragraphs, which can overwhelm the readers. You can break down complex data into manageable sections in order to enhance readability, and for this, you can use the following strategies:
Highlight key findings succinctly through bullet points and numbered lists.
You can make subheadings to structure the discussion around specific data subsets.
Use the technique of short sentences and convey each idea per sentence for clarity.
Give a gist and provide a brief overview before diving into details.
If you compartmentalise all the intricate information, it benefits your readers to absorb data progressively and saves them from struggling with an impenetrable information overload.
Without Sacrificing Accuracy, use Simplified Language
Precision is the most demanding thing in academic writing, but being precise nowhere means that you need to employ language that is overly complete. You should use straightforward terminology with the consideration of not diluting the meaning, and you can replace convoluted phrasing.
Example:
Convoluted Language: The dataset exhibits a statistically significant positive correlation at a p-value of 0.001, signifying a robust association between independent and dependent variables.
Simple Language: The data shows a strong positive relationship confirmed by a highly significant p-value (0.01).
Convoluted Language: The findings demonstrate a substantial divergence from, previously established theoretical paradigms.
Simple Language: The results differ significantly from earlier theories.
You will realise that your data interpretation has become accessible yet academically rigorously prioritises clarity over verbosity.
Within the research, Narrative Contextualise Data
There is a lack of interpretive depth in raw data when it is presented in isolation. How the data is connected to broader academic discussions and why it matters must be understood by the readers.
The best practice for implementing the technique of contextualisation:
To highlight the significance, you can compare your findings with existing literature.
Relate with real-world applications and case studies the statistics.
Explicate what specific reveals and why they were chosen
To demonstrate practical implications, you can use hypothetical scenarios
To prevent the reader from inferring the meaning independently, a well-contextualised dataset works as a guide for the readers through its significance.
Make Use of Data Storytelling Techniques
If numbers are framed correctly, they tell a story. The technique of data storytelling integrates narrative structure with empirical evidence, ensuring that data is not merely reported but woven into engaging academic arguments.
Effective Data Storytelling Elements
In the introduction, present the research question and dataset context
Within the data, try to identify a problem gap or unexpected trend
The findings should be explained in a manner that is compelling and structured.
In conclusion, relate insights to broader implications or future research.
It makes writing more persuasive and impactful if you embed it with a narrative arc instead of treating data as a static entity.
Transparently Address limitations
Obscuring limitations nowhere means simplifying data. Academic integrity is strengthened by cognising methodological shortcomings, potential biases, and data constraints. It demonstrates critical engagement with research findings.
Example:
Do not state: The survey responses indicate a conclusive trend.
State: While survey responses suggest a clear trend, the sample size is limited, and further research is required to confirm generalisability.
Your academic paper maintains credibility and objectivity if you present balanced interpretations.
Final Thoughts
It does not dilute the meaning if you simplify the data. The purpose of simplifying the data is only to enhance clarity, accessibility, and impact. Even intricate datasets can be transformed into compelling academic insights by the researchers if they employ structured methodologies leveraging visual aids and craft cohesive narratives.
Before starting the research, no matter if you are working in scientific research, social sciences, business analytics, or the humanities, you need to master the art of simplifying the data. If you have mastered this art of data simplification, it would be beneficial for your research, as clarity, precision, and intellectual depth will resonate in your academic papers.
Key Takeaways:
Before presenting the data, you should define the core message
For intuitive understanding use graphs, charts, and tables
For conceptual clarity, employ analogies and metaphors
Using lists and subheadings breaks data into manageable sections
Without compromising accuracy, use simplified language
Within the broader research discourse contextualise findings
To make findings engaging and incorporate data storytelling
To maintain integrity, transparently acknowledge limitations
Scholars can easily elevate their academic writings if they master these principles, and it ensures that even the most complex datasets engage, inform, and inspire their readership.
Comentários